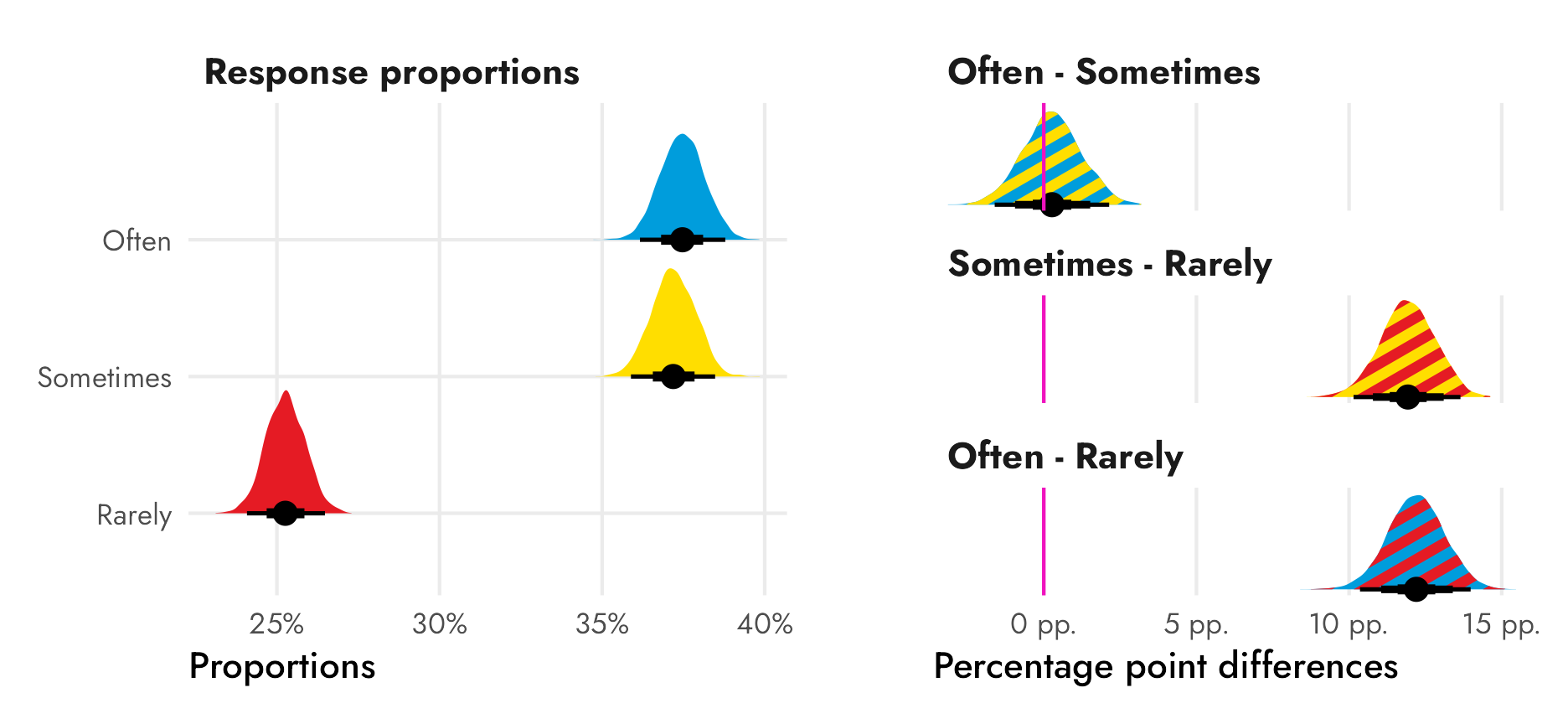
.magic-block { font-style: normal; user-select: none; padding: 0 0.1em 0 0.15em; line-height: 1; font-size: 120%; } I’ve been working on converting a couple of my dissertation chapters into standalone articles, so I’ve been revisiting and improving my older R code.